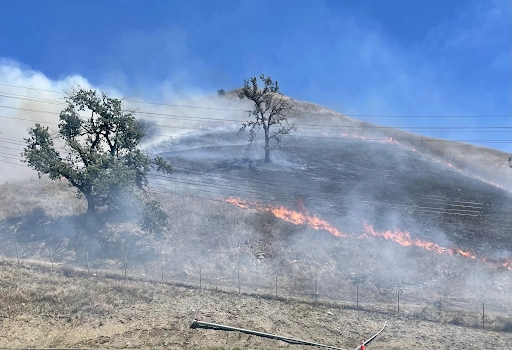
Taking Aim at the Biggest Wildfire Risk
Originally published on Energy Central
A utility’s wildfire plan must include numerous technology elements to effectively reduce wildfire risk. The list of solutions to consider when determining which will help them best is long. Drones. Satellite imagery. LIDAR and geospatial data. Sophisticated weather modeling and forecasts. Grid hardening strategies. Most of these solutions address just a single piece of the wildfire risk mitigation puzzle when used on their own. But when leveraged in combination, they provide valuable mitigation capabilities.
Line sensing is a grid technology often used for improving situational awareness during wildfire events. But when advanced analytics and machine learning are applied to line sensor data, it’s a powerful tool that directly addresses a utility’s biggest wildfire risk – igniting wildfires with the power system.
While no single solution can comprehensively address the threat of wildfire, a solution like line sensing and advanced analytics offers two impactful sets of wildfire risk and reliability benefits:
- The ability to predict and preempt outages
- Improved system visibility and situational awareness
What follows is a brief explanation of how real-time, on-the-wire data from line sensors, advanced analytics, and machine learning come together, as with the Sentient Energy MM3ai System, to address some of utilities’ biggest wildfire risk and reliability concerns.
Outage Prediction to Mitigate the Biggest Risk
Top of mind for every distribution grid operator with a service territory in wildfire prone regions is the risk of utility caused ignition. Outages linked to equipment failure and vegetation contact are among the leading types of permanent faults that cause wildfire ignition.
Intelligent line sensors with advanced analytics and machine learning now make it possible to predict these outages with a high degree of probability.
Here’s how —
Outages associated with equipment failure and vegetation encroachment are often preceded by precursor anomalies before a fault occurs. But anomalies, small deviations of current and voltage waveforms, are too small and short in duration to be detected by protection equipment and cause reclosing or permanent faults.
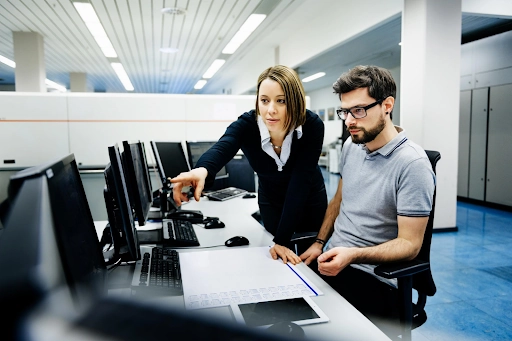
Sentient Energy’s MM3ai line sensor, however, captures data on these anomalies happening on overhead feeders. And when the anomaly data is classified through a cloud-based analytics application, precursor anomalies indicative of equipment failure and vegetation contact can be identified.
Feeder segments with a high probability of impending outage based on precursor anomaly levels are communicated via daily reports. These reports enable the utility to inspect and address the problem before an outage happens, preventing risk of possible ignition.
Equipment Failure Outage Predictions
In the case of an equipment failure prediction, the analytics engine identifies numerous precursor anomalies that indicate a high probability of an impending failure. A utility field inspector is then alerted to the problem via daily reporting so they can investigate and identify the issue on the target feeder segment. Examples of equipment problems that might be visually identified upon inspection include: a broken insulator pin, a cracked cut-out, and a lightning arrestor with detached wires.
Once the issue is confirmed, prompt repair is needed to preempt the likely outage. For the best chance of successfully preempting an outage, total inspection and repair time of one to two days from time of the alert is necessary.
Vegetation Contact Outage Predictions
The process for predicting and preempting an outage caused by vegetation line contact is like that for equipment failure predictions, except that the preemptive action typically involves tree trimming rather than equipment repair.
A prediction of an impending vegetation encroachment outage due is typically communicated to a utility’s vegetation management contact. After the indicated feeder segment is inspected and the vegetation contact issue confirmed, a vegetation management crew must spot trim the vegetation to prevent the outage.
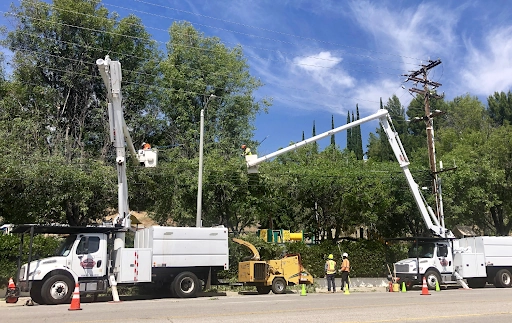
As with equipment failure prediction, a one-to-two-day total response time after the precursor anomalies have been reported is needed for best outage prevention and wildfire risk mitigation results.
Situational Awareness and Fault Location Benefits
In addition to the significant value of outage predictions related to equipment failure and vegetation contact, a line sensing and analytics solution offers several important grid visibility and situational awareness benefits.
When utilities must turn off protection schemes and disable reclosers in wildfire risk areas on high alert days, line sensing provides grid operators valuable system visibility where they would otherwise have little or none.
Disabled reclosers make locating faults more difficult and time consuming which is particularly problematic during periods of high wildfire threat. With line sensors operating at feeder quarter points, crews only need to patrol one quarter of the feeder, enabling reduced patrol time, quicker fault location, and shorter outage durations.
Utilities can also leverage intelligent line sensing for two more system visibility related benefits. The first being — anomaly and fault data from line sensors deployed in rugged or outlying terrain with challenging access can be used to remotely direct high resolution wildfire cameras to zero in on feeder areas that warrant visual inspection. And the second being — waveforms captured by line sensors during faults allow engineers to verify that protection schemes are working properly, as shown below:
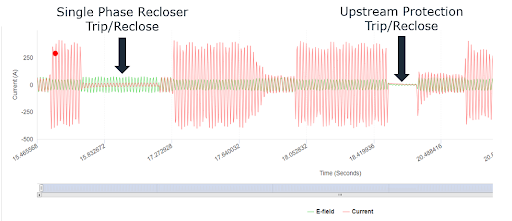
A New Opportunity to Take Aim at the Biggest Risk
When technologies improve, new opportunities emerge, as is the case with intelligent line sensing and advanced analytics.
The combination of recent advances in edge computing, cellular communications, and cloud-based analytics provides utilities with a new, cost-effective way to aggressively reduce their biggest wildfire risk — by predicting two leading types of outages linked to wildfire ignition caused by the power system. And with the same easy to deploy, line-powered solution that enables outage predictions, they can also improve reliability with system visibility, situational awareness, and reduced outages.
To be broad reaching and effective, utility wildfire risk mitigation plans must include a variety of technology solutions. Now that predicting and preempting outages is technically possible, line sensing and advanced analytics is a must have multi-pronged solution component for any comprehensive utility wildfire risk mitigation plan.
Contact us to learn more about how Sentient Energy’s MM3ai System blends the innovation of predictive analytics and machine learning applied to real-time data with the practical value of multiple, important use cases and benefits.