Address complex challenges with advanced analytics and line sensing
Sentient Energy’s MM3ai System uses a powerful combination of technologies to address complex power deliver challenges. The system combines advanced analytics, line sensors, and cloud-based software to provide utilities with reports containing predictive indicators of impending outages, detection of elevated harmonics levels, abnormal fault conditions, and automated distance to fault estimates. Plus, the system provides fault detection and load data from more grid locations.
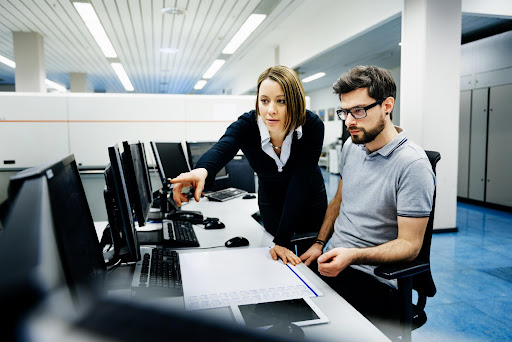
Key Features of the MM3ai System
The MM3ai System is a complete package designed for utilities to get started predicting impending outages and measuring harmonics levels while also leveraging the solution’s fault detection & location and load monitoring capabilities. The system generates daily or weekly reports and includes sensors, software, cellular fees and support including:
- Ninety-six MM3ai Line Sensors for quarter point visibility on eight feeders
- Ample Analytics Platform with managed cloud deployment
- Cellular fees
- Deployment and ongoing support, including report training and one-hour monthly meetings
- Software updates
- Warranty
Accelerate Grid Modernization with the MM3ai System
Analytics Capabilities
The MM3ai System analytics enable grid modernization and operations teams to predict and preempt outages for reliability improvements, cost savings, and wildfire risk mitigation. Analytics also help power quality and protection engineers identify feeder segments with elevated harmonics levels and abnormal fault events. And, MM3ai System analytics integrates fault information with power flow models to provide more accurate and timely estimates of distance to faults.
Advanced Software Platform
Ample Analytics, with its flexible architecture, can be deployed either on-premises or in the cloud. It also supports a hybrid approach in which utilities run certain components, such as analytics modules, in the cloud, while securely integrating line sensor and grid edge control data with on-premises operational technology (OT) systems like SCADA, ADMS, or OMS.
High Resolution Data Capture
The MM3ai sensors capture data at high resolution, 130 samples per cycle, orders of magnitude better than most SCADA devices. At this resolution analytics can detect more minor disturbances like precursor anomalies to predict and preempt outages. In addition, higher resolution data can be used to address other complex grid issues like determining the causes of elevated harmonics, identifying abnormal faults, and estimating distance to faults.
Amplify Predictive Capabilities with the MM3ai
Optimize Vegetation Management
Vegetation management is often the single largest line item of utility operations and maintenance budgets, even exceeding $100 million annually for many larger utilities. Vegetation management is not new to utilities – optimize your vegetation management by using the MM3ai System and provide a new way to better prioritize vegetation management work based on outage precursor anomalies.
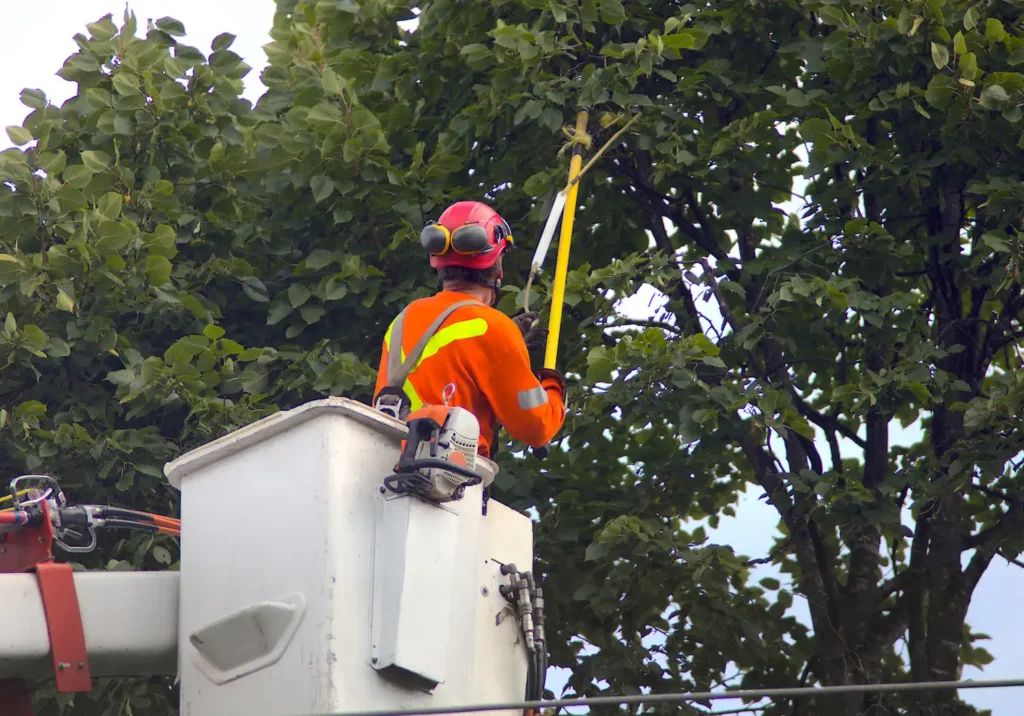
Predict and Preempt Outages
Line sensors detect anomalies and capture waveforms which are then run through analytics models to identify precursors to permanent faults for equipment failure and vegetation contact with conductors. The MM3ai System generates daily reports with precursor anomalies by sensor location to enable data-driven proactive inspections and equipment repairs or tree trimming.
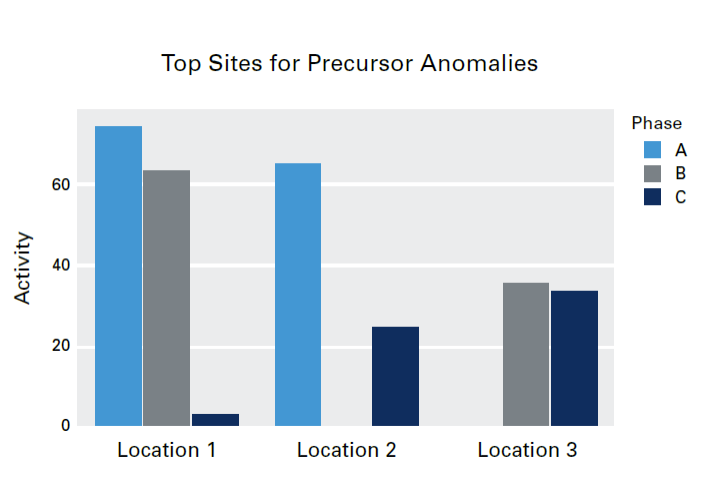
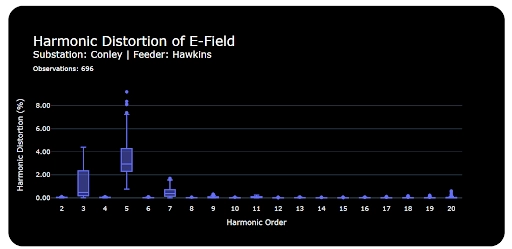
Harmonics Measurement
Sentient Energy MM3ai line sensors capture anomalies in waveforms and with advanced analytics, levels of harmonic distortion can be determined. The system generates weekly reports that provide harmonics measurements for each distribution feeder so utilities can identify elevated harmonics levels, isolate sources of harmonics problems, and take action to address them.
Fault Insights and Automated Fault Location
The MM3ai System also provides two additional analytics capabilities. First, the system generates a Fault Insights report for protection engineers identifying fault events and patterns that are abnormal based on duration, magnitude, or frequency of occurrence. Second, the system provides operations teams more accurate and timely estimates of faulted locations on a geospatial view of the feeder in near real-time following a fault. These location estimate help crew accelerate fault finding.
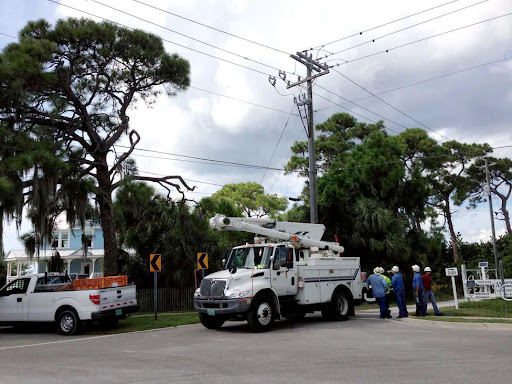