Case Study
Leveraging the Cloud and Artificial Intelligence to Preempt Power Outages
The Challenge
Aging infrastructure, much of it 100 years old or more and nearing end-of-life, presents utilities with a variety of grid reliability and resilience challenges. And a confluence of extreme weather, wildfire events, and increasing electrification needs serve to make reliability and resilience challenges even more pronounced. For many utilities, operations and maintenance budget constraints have limited their ability to make the grid modernization investments needed to keep up with customer expectations for reliable power delivery.
Traditionally, utilities have relied upon reactive switching and protection to manage system faults and reduce outage times, only seeking to remedy the initial cause of the fault after an outage. In the case of an outage caused by equipment failure, this reactive approach may lead to additional costs to the utility when it leads to the need for equipment replacement rather than repair. Add to that, lower customer satisfaction and lost revenues.
The Solution
Utilities typically use a combination of complementary solutions to improve grid reliability and resilience, with many leveraging intelligent line sensors for increased system visibility, fault detection and location, and load monitoring. When line sensing is combined with cloud-based advanced analytics and machine learning, the solution helps utilities predict and preempt permanent faults due to equipment failures and vegetation contact.
Intelligent line sensors are autonomous connected devices that monitor and record disturbance activity on the grid. When this disturbance activity data is further analyzed with a cloud-based analytics platform and outage precursor anomalies identified, a utility can evolve from reactively addressing faults to proactively mitigating the cause for a fault and thus, preempting outages.
This powerful approach provides new ways to improve key performance metrics significantly and simultaneously, such as SAIDI, SAIFI, and MAIFI, by informing the utility’s operators of a future outage before customers experience an interruption. This is achieved by deploying Sentient Energy’s MM3ai System comprised of MM3ai intelligent line sensors and a cloud-based advanced analytics module powered by AWS. This state-of-the-art system enables utilities to embark on a paradigm shift towards proactive outage mitigation and grid management.
Field Deployment Example
A leading investor-owned utility in the Midwest recently leveraged intelligent line sensors and advanced analytics to preempt outages due to equipment failure showcasing the power of predictive analytics for reliability and resilience improvement. With multiple intelligent line sensors deployed on a feeder, one of the line sensors, located just downstream of a hydraulic recloser, captured multiple low amplitude (<10A) disturbance signatures. These disturbances were classified by Sentient Energy’s machine learning models as precursor anomalies, correlated with active equipment failure. The observed activity downstream of this sensor site resulted in the sensor site location escalating to a “Watch” status.
Approximately a week later, similar precursor anomalies resurfaced, leading to a second escalation at the sensor site, a “Warning” status. Sentient Energy engineers reviewed the escalation with the utility, drawing attention to the imminence of an equipment failure. Roughly two weeks after the initial Watch indication and with the evidence gathered over that time, the utility dispatched a crew to conduct a field investigation. The crew initially found no clear evidence of equipment failure in their exploration despite the evidence observed in the electrical disturbances.
The failing equipment did not remain hidden for long though. A few days after the field inspection, the line sensor reported a single-phase fault with a momentary interruption cleared by a hydraulic recloser operation. This event was not visible to the utility prior to the line sensor installation due to the lack of SCADA visibility at this site. Based on the fault current captured by the sensor, however, potential fault locations downstream of the device were identified. The utilities initiated a second field investigation occurred a few days after the momentary fault, where the crew identified a cracked fuse cutout at the second identified location. The cutout was replaced and the precursor anomalies for this event ceased after the repairs were made.
This example highlights how the MM3ai System, leveraging advanced electrical disturbance classification algorithms and predictive outage models, can identify an impending failure and alert the utility prior to a sustained outage. Precursor anomalies do not necessarily occur at regular intervals, but rather when specific environmental or electrical conditions are met. In this instance, significant precursor anomalies occurred on four separate days, followed by a fault. None of the precursor anomalies prior to the fault were detectable by traditional distribution protective devices given the subtleties.
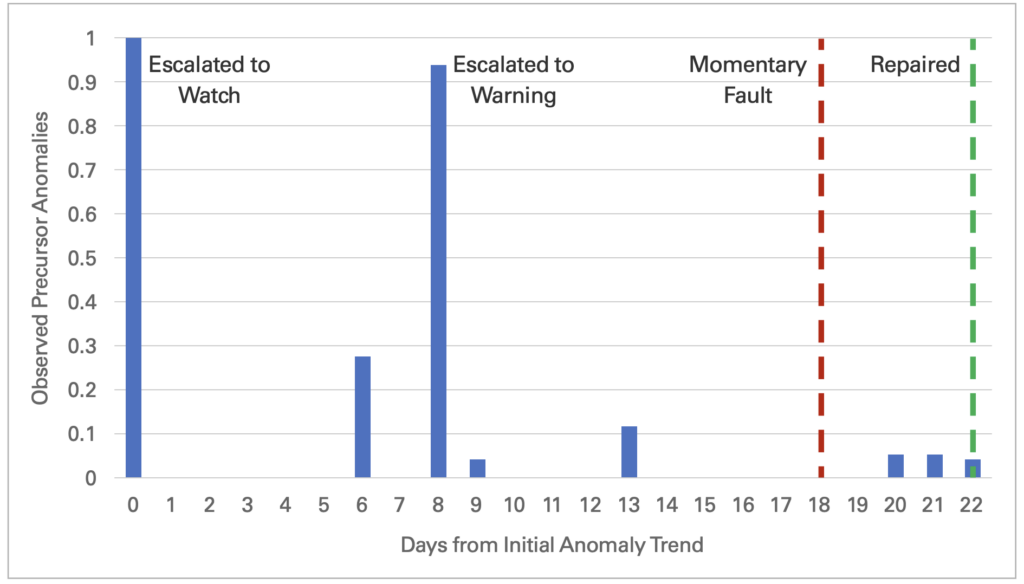
Conclusion
Advance notification via Sentient Energy’s MM3ai System reporting and proactive actions taken by the utility prevented a sustained outage on the protective zone where the failing cutout was located. Assuming the feeder had a 300-minute disruption (based on historical records) the prevented outage would have caused an estimated 42,000 Customer Minutes Interrupted (CMI) for this specific failure. Ultimately, the ability to prevent outages at-scale and shift towards proactive fault management will enable prolonged asset performance, significant reliability improvement, and superior customer satisfaction. This is the promise of predicting and preempting outages, made possible by advanced analytics, machine learning, and digitalization, and fulfilled in the utility space.
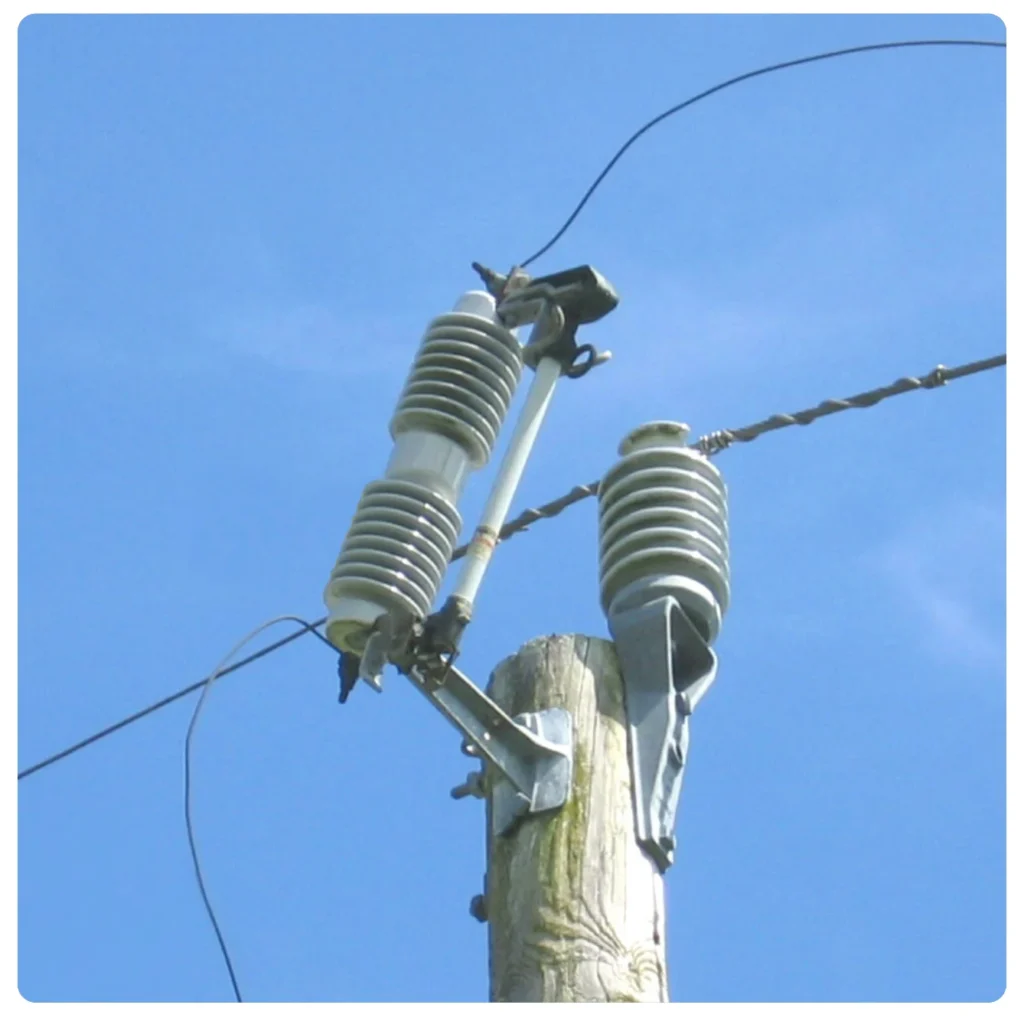
Image of a fused cutout: https://commons.wikimedia.org/w/index.php?curid=19412790